本文转载至知乎ID:Charles(白露未晞)知乎个人专栏
下载W3Cschool手机App,0基础随时随地学编程>>戳此了解
导语
本文转载至知乎ID:Charles(白露未晞)知乎个人专栏
下载W3Cschool手机App,0基础随时随地学编程>>戳此了解
昨天在看GitHub上深度学习方面stars较高的开源项目,于是发现了这个有趣的内容:
使用深度强化学习破解Flappy Bird游戏(深度Q-学习)。
相关文件
百度网盘下载链接: https://pan.baidu.com/s/19LgDHq0V3IpE1K5sfuug2g
密码: tqus
参考文献
内容主要参考自GitHub开源项目:
Using Deep Q-Network to Learn How To Play Flappy Bird
链接:
https://github.com/yenchenlin/DeepLearningFlappyBird
原理简介
此项目参考了深度增强学习中的深度Q学习算法,并表明了此学习算法可以推广到破解Flappy Bird游戏当中。也就是说,项目是利用了Q-learning的变体进行训练的,其输入是原始像素输出是估计之后行动的数值函数。
PS:
若对深度强化学习感兴趣,公众号相关文件中也提供了一篇名为Demystifying Deep Reinforcement Learning的论文供大家学习,这也是原作者强烈推荐的论文。
网络架构:
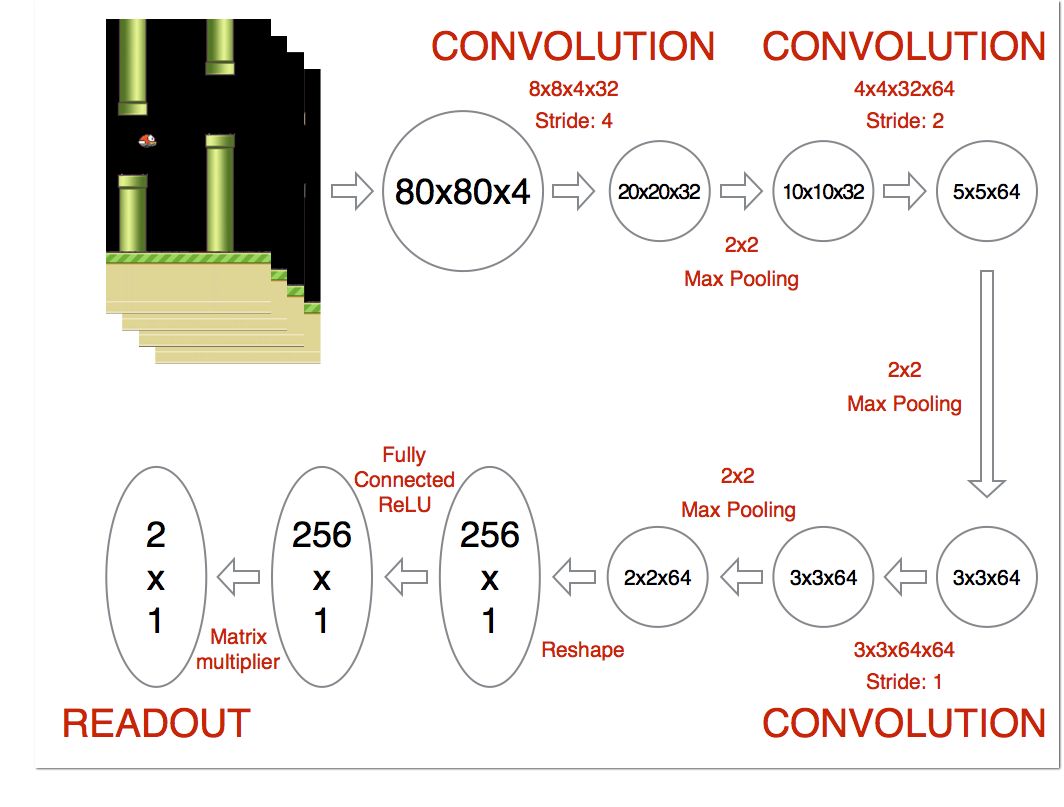
在此之前的预处理为:
(1)灰度化图像;
(2)图像大小调整为80×80;
(3)每4帧画面堆叠成一个80x80x4输入数组。
网络最终输出结果为2×1的矩阵,用以决定小鸟是否行动。(也就是是否按屏幕咯~~~)
测试环境
电脑系统:Win10
Python版本:3.5.4
Python相关第三方库:
TensorFlow_GPU版本:1.4.0
Pygame版本:1.9.3
OpenCV-Python版本:3.3.0
具体配置细节请参考相关网络文档!!!
运行演示
命令行窗口进入DeepLearningFlappyBird文件夹输入py -3.5 deep_q_network.py回车运行即可:

结果如下:
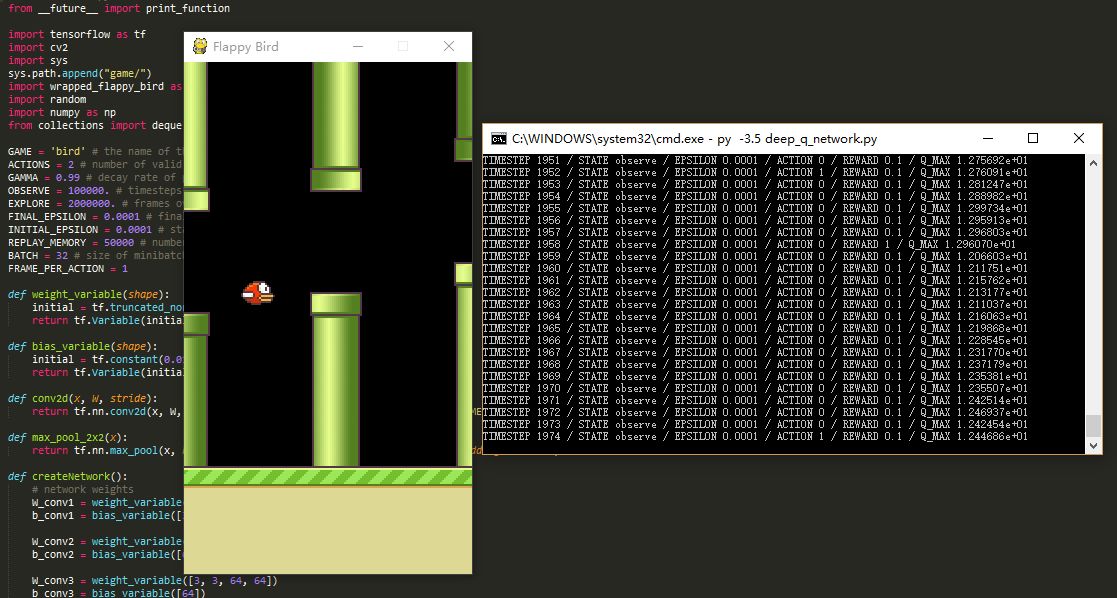
更多参考文献
(1) Mnih Volodymyr, Koray Kavukcuoglu, David Silver, Andrei A. Rusu, Joel Veness, Marc G. Bellemare, Alex Graves, Martin Riedmiller, Andreas K. Fidjeland, Georg Ostrovski, Stig Petersen, Charles Beattie, Amir Sadik, Ioannis Antonoglou, Helen King, Dharshan Kumaran, Daan Wierstra, Shane Legg, and Demis Hassabis. Human-level Control through Deep Reinforcement Learning. Nature, 529-33, 2015.
(2) Volodymyr Mnih, Koray Kavukcuoglu, David Silver, Alex Graves, Ioannis Antonoglou, Daan Wierstra, and Martin Riedmiller. Playing Atari with Deep Reinforcement Learning. NIPS, Deep Learning workshop.
(3)Kevin Chen. Deep Reinforcement Learning for Flappy Bird Report | Youtube result.
链接:
https://youtu.be/9WKBzTUsPKc
(4)https://github.com/sourabhv/FlapPyBird
(5)https://github.com/asrivat1/DeepLearningVideoGames